Verticalization of Large Language Models: A Complete Guide
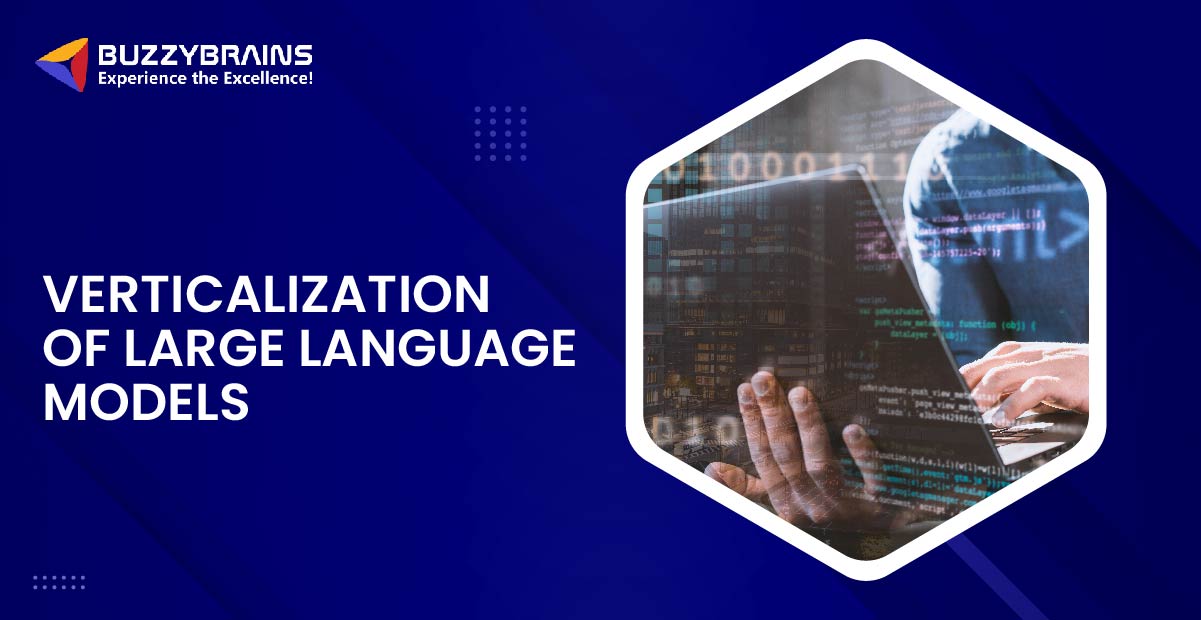
As artificial intelligence (AI) technology advances, large language models (LLMs) have become essential tools in various fields. These models, like OpenAI’s GPT-4, have transformed the way we interact with technology, providing a foundation for chatbots, content generation, and more. However, the broad scope of these general-purpose LLMs may not always suit the specific needs of each industry.
This is where verticalization comes into play. By tailoring LLMs to specific industries, businesses can unlock unique insights and address specialized tasks effectively. In this guide, we’ll dive into the concept of verticalizing LLMs and explore its benefits, challenges, and future potential.
- What are Large Language Models (LLMs)?
- What is Verticalization of LLMs?
- Steps in Verticalizing LLMs
- Industries Leveraging Verticalized LLMs
- Benefits of Verticalizing LLMs
- Challenges in Verticalizing LLMs
- Techniques and Approaches for Verticalization
- Use Cases for Verticalized LLMs
- Future Trends in the Verticalization of LLMs
- FAQs about Verticalization of Large Language Models
- Conclusion
- Bring the Benefits of Verticalized AI to Your Business – Partner with BuzzyBrains!
What are Large Language Models (LLMs)?
Large Language Models, or LLMs, are AI systems trained on massive datasets of text to understand and generate human-like language. These models use advanced algorithms to predict the likelihood of word sequences, enabling them to complete sentences, answer questions, and even write essays. With billions of parameters, LLMs like GPT-3 and GPT-4 can process complex language structures, making them versatile for various applications.
LLMs have the capability to transform text-based tasks across different fields. However, their general-purpose nature often lacks the depth required for specialized industry tasks. This is where verticalized models step in to fill the gap, providing precise language processing tailored to specific sectors.
What is Verticalization of LLMs?
Verticalization of LLMs refers to the process of customizing the large language models for specific industries. Instead of creating a “one-size-fits-all” model, verticalized LLMs are trained with industry-specific data, jargon, and scenarios. This customization allows these models to perform better in specialized tasks compared to generalized LLMs.
For example, a healthcare LLM can be verticalized to assist in medical diagnosis, while a legal LLM can analyze contracts and specific case laws. By focusing on a single industry, verticalized LLMs can better understand context, reduce error rates, and enhance user experiences.
Steps in Verticalizing LLMs
To create a verticalized LLM, several essential steps must be followed. Each step ensures that the model is both relevant and accurate for the target industry.
- Define Goals and Scope: Determine the industry-specific tasks and outcomes required from the verticalized model.
- Collect Industry-Specific Data: Gather data unique to the industry, including jargon, terminology, and case studies.
- Fine-Tune the Model: Adjust the general LLM using industry data through fine-tuning to improve relevancy.
- Validate and Test the Model: Conduct thorough testing on industry tasks to ensure accuracy and reliability.
- Implement Continuous Improvement: Regularly update the model with new data and advancements in the industry.
Each of these steps is crucial in developing a model that not only performs well but also remains aligned with industry trends and needs.
Industries Leveraging Verticalized LLMs
Many industries are now adopting verticalized LLMs to solve specific challenges unique to their domains. Here are some key industries:
- Healthcare
- Finance
- Legal
- Retail and E-commerce
- Education
- Manufacturing
- Marketing and Advertising
- Travel and Hospitality
Each industry benefits from specialized models that can interpret and analyze data within a relevant context, resulting in improved efficiency and customer satisfaction.
Benefits of Verticalizing LLMs
Verticalized LLMs provide many advantages, particularly in enhancing the performance of AI models for industry-specific tasks. Key benefits include:
- Increased Relevance: Tailored language models provide more accurate responses relevant to the industry.
- Enhanced Accuracy: Verticalized LLMs reduce error rates in complex, industry-specific tasks.
- Better Compliance: Specialized models help industries stay compliant with regulations.
- Improved Productivity: Streamlined workflows improve productivity by automating repetitive tasks.
- Cost Efficiency: Industry-specific models may reduce the need for human intervention, saving costs.
By addressing unique needs within industries, verticalized LLMs optimize processes and provide businesses with valuable, actionable insights.
Challenges in Verticalizing LLMs
Despite their benefits, creating a verticalized LLM comes with several challenges. Here are a few key hurdles:
- Data Availability: Finding large, high-quality datasets in specialized fields can be challenging.
- Technical Complexity: Customizing models requires both AI expertise and industry knowledge.
- Privacy and Security: Industries like healthcare and finance face strict data privacy regulations.
- High Development Costs: Developing and maintaining specialized models can be costly.
- Potential Bias: Industry-specific data may introduce biases that impact model fairness.
Addressing these challenges is essential to create effective, ethical, and practical verticalized LLMs for long-term use.
Techniques and Approaches for Verticalization
Verticalizing LLMs involves several techniques to ensure they perform accurately within specific fields. Here are some of the primary approaches:
- Fine-Tuning: Retrain the model with domain-specific data.
- Prompt Engineering: Develop specific prompts to improve responses for industry tasks.
- Knowledge Graph Integration: Use industry knowledge bases to enhance model understanding.
- Data Augmentation: Increase the model’s dataset with synthetic examples or underrepresented scenarios.
- Continuous Training: Regularly update the model to keep it aligned with industry advancements.
These approaches contribute to creating a model that can effectively address industry-specific requirements and challenges.
Use Cases for Verticalized LLMs
Verticalized LLMs are making a significant impact across various industries. Here are some popular use cases:
- Healthcare: Assisting doctors with diagnostic information and patient records analysis.
- Finance: Providing insights for financial forecasting and compliance checks.
- Legal: Automating contract review and case law analysis.
- Retail: Generating personalized recommendations and customer support responses.
- Education: Supporting personalized learning by adapting content to student needs.
These use cases showcase how verticalized LLMs can transform operations, boost efficiency, and enhance outcomes within each industry.
Future Trends in the Verticalization of LLMs
As the field of AI grows, verticalization is expected to become more sophisticated. Some emerging trends include:
- Further Specialization: Models will focus on subfields and mission-critical tasks within industries, like pediatric healthcare & more.
- Collaborative Development: AI and industry experts will increasingly collaborate on verticalized models.
- Privacy-Enhancing Techniques: Innovations like federated learning will address data privacy concerns.
- Open-Source Verticalized Models: Shared resources and open-source models will promote wider adoption.
- Bias Mitigation: Continued research to reduce industry-specific biases will enhance model fairness.
These trends suggest that verticalized LLMs will continue to evolve, offering even more precise and ethical solutions for specific domains.
FAQs about Verticalization of Large Language Models
To address common questions, here are some frequently asked questions about the verticalization of LLMs.
Q1. Are verticalized LLMs more cost-effective for businesses?
Yes, verticalized LLMs can reduce operational costs by streamlining industry-specific processes and improving accuracy in automated tasks, which can lower the need for human intervention.
Q2. How are verticalized LLMs trained differently than general LLMs?
Verticalized LLMs are trained using industry-specific data and scenarios, allowing them to understand context and terminology unique to a particular field.
Q3. What is the role of industry-specific data in verticalizing LLMs?
Industry-specific data is crucial for verticalization, as it helps the model learn and interpret field-specific language, reducing errors and improving relevance.
Q4. How does verticalization impact the scalability of LLMs?
While verticalization can increase relevance, it may limit the model’s generalizability, which can impact scalability outside the targeted industry.
Q5. What skills are needed to create a verticalized LLM?
Developing verticalized LLMs requires expertise in AI, natural language processing, and a strong understanding of the target industry’s specific needs.
Conclusion
Verticalization of large language models is a game-changer for businesses looking to harness AI for industry-specific applications. By customizing LLMs, companies can achieve greater accuracy, improve compliance, and increase efficiency within their respective fields. However, verticalization does come with challenges, such as high development costs and technical complexity.
Despite these challenges, the benefits of verticalized LLMs make them a powerful tool in today’s competitive landscape. With continued advancements, these specialized models have the potential to redefine industry standards and drive innovation.
Bring the Benefits of Verticalized AI to Your Business – Partner with BuzzyBrains!
Is your business ready to leverage the advantages of verticalized AI? At BuzzyBrains, we specialize in developing customized, industry-specific LLMs that can transform operations and enhance productivity. Whether you’re in healthcare, finance, retail, or another field, our expertise can help you get the most out of AI.
Get in touch with BuzzyBrains today to learn more about how we can bring the power of verticalized AI to your business!