Best Big Data Analytics Tools: A Comprehensive Guide to Choosing the Right Tools
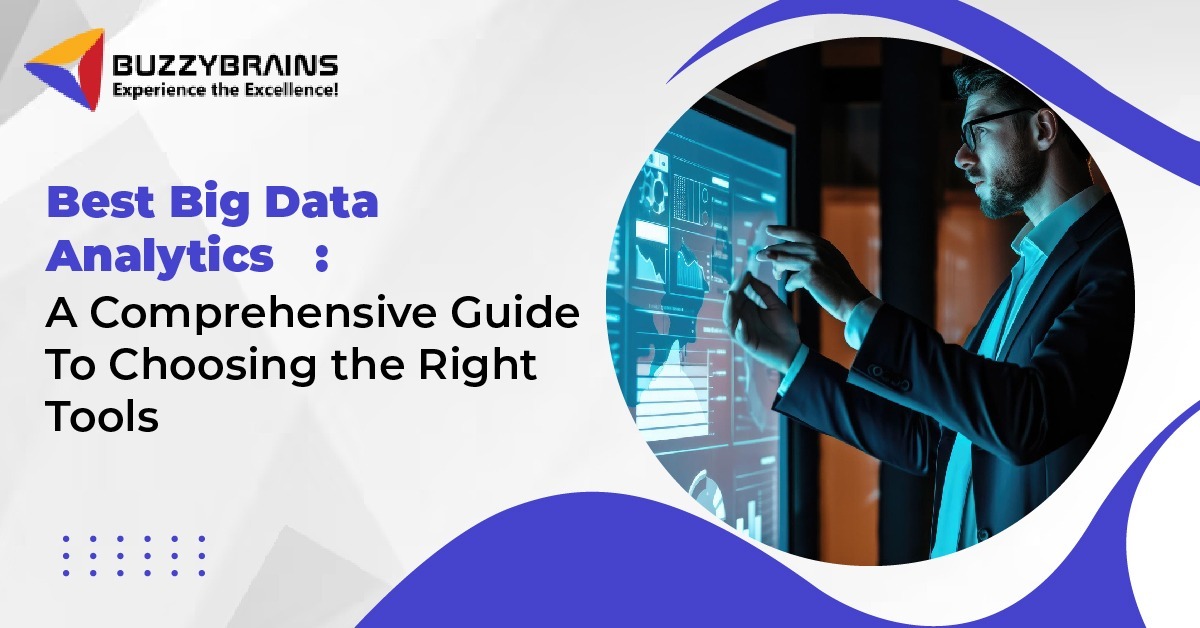
Big data analytics tools have become indispensable in today’s data-driven world. With massive amounts of data generated every second, businesses need robust tools to extract valuable insights and make informed decisions. This comprehensive guide will delve into the key factors to consider when choosing big data analytics tools, highlight the top tools in 2024, provide steps to choose the right tool and showcase real-world examples.
- What are Big Data Analytics Tools?
- Factors to Consider When Choosing Big Data Analytics Tools
- Top 10 Best Big Data Analytics Tools in 2024
- Choosing the Right Big Data Analytics Tool
- Real-world Examples of Companies Using Big Data Analytics Tools
- FAQs about How to Choose Big Data Analytics Tools
- Conclusion
- Supercharge Your Data Insights Today with BuzzyBrains!
What are Big Data Analytics Tools?
Big data analytics tools encompass a range of software and platforms designed to process, analyze, and visualize vast volumes of structured and unstructured data. These tools leverage advanced algorithms, machine learning, and artificial intelligence to uncover patterns, trends, and correlations that are otherwise challenging to discern manually.
Factors to Consider When Choosing Big Data Analytics Tools
Selecting the appropriate big data analytics tools can have a profound influence on the effectiveness and outcomes of your data-centric endeavours. Here are numerous key factors to take into account when navigating this decision-making process:
1. Scalability
Scalability refers to a tool’s ability to handle increasing data volumes and processing demands without compromising performance. A scalable tool should seamlessly accommodate growing datasets and user loads while maintaining responsiveness.
2. Data Sources
Consider the types and sources of data your organization deals with. Ensure the chosen tool can integrate with various data sources such as databases, cloud storage, IoT devices, social media platforms, and more.
3. Performance
Evaluate the tool’s processing speed, accuracy of analysis, and real-time capabilities. High-performance tools can process complex queries swiftly and deliver insights in a timely manner, crucial for agile decision-making.
4. Ease of Use
User-friendliness is vital for widespread adoption within your organization. Look for intuitive interfaces, customizable dashboards, and comprehensive documentation or training resources to facilitate ease of use.
5. Integration
Check compatibility with existing systems, applications, and workflows. Seamless integration ensures smooth data flows and enhances collaboration across departments.
6. Cost
Consider both upfront costs (licensing, implementation) and ongoing expenses (maintenance, support, upgrades). Opt for a tool that offers value for money and aligns with your budgetary constraints.
7. Security
Data security and compliance are paramount. Ensure the tool implements robust security measures such as encryption, access controls, and compliance with industry standards (e.g., GDPR, HIPAA).
By carefully considering these factors, you can select a big data analytics tool that meets your organization’s specific needs and empowers you to derive actionable insights from your data effectively.
Top 10 Best Big Data Analytics Tools in 2024
Selecting the most suitable big data analytics tools hinges on tailoring your choice to match your unique needs and demands. Here are 10 of the highly favoured tools in 2024, each renowned for its distinct strengths and capabilities:
1. Hadoop
Hadoop is an open-source framework for distributed storage and processing of big data.
Key Features: Scalability, fault tolerance, MapReduce programming model.
Use Cases: Large-scale data processing, batch analytics, data lake implementations.
Pros: Cost-effective, community support, ecosystem of tools.
Cons: Steep learning curve, infrastructure requirements.
2. Apache Spark
Apache Spark is a fast and general-purpose cluster computing system.
Key Features: In-memory processing, advanced analytics, streaming data support.
Use Cases: Real-time analytics, machine learning, graph processing.
Pros: High performance, ease of use (APIs in Java, Scala, Python), versatile.
Cons: Resource-intensive, complexity in managing clusters.
3. Tableau
Tableau is a data visualization tool that helps users create interactive and shareable dashboards.
Key Features: Drag-and-drop interface, real-time analytics, extensive data connectors.
Use Cases: Business intelligence, data storytelling, visual exploration of datasets.
Pros: User-friendly, robust visualization options, strong community support.
Cons: Pricing for enterprise editions, limited advanced analytics capabilities.
4. Microsoft Power BI
Microsoft Power BI is a business analytics tool for creating interactive reports and dashboards.
Key Features: Data modelling, AI-driven insights, integration with Microsoft ecosystem.
Use Cases: Data-driven decision-making, self-service analytics, collaboration.
Pros: Seamless integration with Microsoft products, natural language querying, scalable.
Cons: Learning curve for complex data modelling, limited customization options.
5. Google BigQuery
Google BigQuery is a serverless, highly scalable cloud data warehouse for analytics.
Key Features: Managed service, real-time data processing, SQL-based querying.
Use Cases: Data exploration, ad hoc analysis, predictive analytics.
Pros: Scalability, cost-effective (pay-per-query pricing), integration with Google Cloud Platform.
Cons: Query pricing can escalate with large datasets, requiring familiarity with SQL.
6. Apache Flink
Apache Flink is a distributed stream processing framework for real-time analytics.
Key Features: Event time processing, state management, fault tolerance.
Use Cases: Real-time data processing, event-driven applications, IoT analytics.
Pros: Low-latency processing, high throughput, support for event-driven architectures.
Cons: Complex setup for large clusters, limited graphical tooling.
7. Snowflake
Snowflake is a cloud-based data platform for data warehousing and analytics.
Key Features: Data sharing, instant elasticity, automatic scaling.
Use Cases: Data lakes, data warehousing, data sharing across organizations.
Pros: Zero maintenance, multi-cloud support, granular security controls.
Cons: Cost can escalate with usage, learning curve for some advanced features.
8. RedShift
Amazon Redshift is a fully managed, petabyte-scale data warehouse service in the cloud, offered by Amazon Web Services (AWS).
Key Features: Massively parallel processing (MPP), columnar storage, automatic backups, scalable compute and storage.
Use Cases: Business intelligence (BI), data warehousing, large-scale analytics, data lake integration.
Pros: High performance for complex queries, integration with other AWS services, cost-effective scaling, SQL-based interface.
Cons: Requires AWS ecosystem knowledge, can be expensive for smaller workloads, limited availability outside of AWS regions.
9. Databricks
Databricks is a unified analytics platform for big data and machine learning.
Key Features: Apache Spark integration, collaborative workspace, automated ML.
Use Cases: Data engineering, data science, machine learning pipelines.
Pros: Simplified data processing, collaborative environment, scalable infrastructure.
Cons: Pricing based on usage, may require expertise in Spark and cloud platforms.
10. Azure Synapse Analytics
Azure Synapse Analytics is a powerful analytics service provided by Microsoft Azure, designed to handle big data and analytics workloads.
Key Features: Integrated analytics platform, data integration, big data processing, enterprise data warehousing.
Use Cases: Data exploration and analysis, business intelligence (BI), data engineering, real-time analytics.
Pros: Unified analytics service, seamless integration with Azure services, support for multiple programming languages (SQL, Python, Scala), auto-scaling capabilities.
Cons: Requires familiarity with Azure ecosystem, potential cost concerns with large-scale deployments, learning curve for advanced features.
Related Blog: Top Data Engineering Tool & Platforms: A Comprehensive Tool Guide
Choosing the Right Big Data Analytics Tool
Choosing the right big data analytics tool requires a systematic approach to match your organization’s needs with the capabilities of the available tools. Here are steps to evaluate and choose the right tool:
Step 1: Define Requirements
Begin by clearly defining your organization’s goals, data sources, analysis requirements, user roles, and budget constraints. Consider factors such as data volume, complexity, real-time processing needs, and integration with existing systems.
Step 2: Research and Compare
Research different tools based on your requirements. Look for features such as scalability, performance, ease of use, integration capabilities, security measures, and pricing models. Compare multiple tools to understand their strengths and weaknesses.
Step 3: Evaluate Scalability and Performance
Conduct scalability and performance tests with sample datasets or pilot projects. Assess how each tool handles increasing data volumes, concurrent users, complex queries, and real-time processing. Consider factors like processing speed, memory utilization, and response times.
Step 4: Consider Integration and Security
Evaluate each tool’s compatibility with your existing infrastructure, data sources, and applications. Ensure seamless integration to avoid data silos and facilitate data flows across systems. Assess the tool’s security features, including data encryption, access controls, compliance certifications, and audit trails.
Step 5: Total Cost of Ownership (TCO)
Calculate the total cost of ownership (TCO) for each tool, including upfront costs (licensing, implementation, training) and ongoing expenses (maintenance, support, upgrades). Consider the scalability of pricing models and potential return on investment (ROI) in terms of improved analytics capabilities and business outcomes.
Step 6: User Adoption and Training
Consider the ease of use and learning curve for each tool. Evaluate available training resources, documentation, user communities, and vendor support. Choose a tool that can be adopted seamlessly by your teams and offers adequate training and support options.
By following these steps, you can evaluate and choose the right big data analytics tool that aligns with your organization’s goals, technical requirements, budget, and user preferences.
Real-world Examples of Companies Using Big Data Analytics Tools
Here are some compelling real-world examples showcasing how companies are harnessing the power of big data analytics tools to foster innovation and improve decision-making processes:
1. Netflix (Apache Spark):
Netflix, the global streaming giant, relies heavily on big data analytics to personalize content recommendations for its users.
Tool Used: Apache Spark
Use Case: By leveraging Apache Spark’s real-time analytics capabilities, Netflix processes vast amounts of viewer data to understand user preferences, viewing habits, and engagement patterns. This data is then used to generate personalized recommendations, improve content curation, and enhance the overall user experience.
Impact: Netflix’s use of Apache Spark has significantly contributed to its success in retaining subscribers and increasing viewer satisfaction. The platform’s ability to deliver relevant content recommendations has led to higher user engagement, longer viewing sessions, and reduced churn rates.
2. Uber (Hadoop):
Uber, the ride-hailing giant, handles massive amounts of data related to trip bookings, driver-partner information, and customer feedback.
Tool Used: Hadoop
Use Case: Hadoop’s distributed storage and processing capabilities are instrumental in managing Uber’s data-intensive operations. The platform processes real-time location data, calculates optimal routes, performs demand forecasting, and analyzes driver performance metrics to optimize service delivery and pricing strategies.
Impact: By harnessing the power of Hadoop, Uber has improved operational efficiency, reduced wait times for riders, optimized driver earnings, and enhanced overall customer satisfaction. The platform’s data analytics capabilities drive data-driven decision-making across various aspects of the business.
3. Amazon (Google BigQuery):
Amazon, the e-commerce giant, deals with vast amounts of customer transaction data, product inventory, and supply chain information.
Tool Used: Google BigQuery
Use Case: Google BigQuery’s cloud-based data warehouse capabilities enable Amazon to analyze massive datasets quickly and derive actionable insights. The platform supports ad hoc queries, predictive analytics, and data visualization, empowering Amazon’s teams to make data-driven decisions in areas such as inventory management, pricing optimization, and customer segmentation.
Impact: By leveraging Google BigQuery, Amazon has gained valuable insights into customer behavior, market trends, and operational efficiency. The platform’s scalability and real-time querying capabilities have helped Amazon streamline its business processes, improve decision-making agility, and drive revenue growth.
4. Walmart (Tableau and Microsoft Power BI):
Walmart, the retail giant, collects extensive data on customer transactions, inventory levels, sales trends, and supply chain operations.
Tools Used: Tableau and Microsoft Power BI
Use Case: Walmart utilizes Tableau and Microsoft Power BI for data visualization, reporting, and business intelligence. These tools enable Walmart’s teams to create interactive dashboards, analyze sales performance, track inventory metrics, optimize store layouts, and identify market trends. The platforms facilitate data-driven decision-making at various levels of the organization.
Impact: By leveraging Tableau and Microsoft Power BI, Walmart has improved visibility into its operations, enhanced inventory management practices, optimized product assortments, and personalized marketing strategies. The platforms’ intuitive interfaces and robust analytics capabilities have empowered Walmart’s teams to drive operational excellence and deliver superior customer experiences.
These real-world examples demonstrate how leading companies across industries leverage big data analytics tools to gain insights, improve decision-making, enhance operational efficiency, and drive business growth. By harnessing the power of advanced analytics platforms, organizations can unlock the full potential of their data and stay competitive in today’s data-driven landscape.
FAQs about How to Choose Big Data Analytics Tools
Q1. How do I compare the performance of different analytics tools?
Compare processing speeds, memory usage, scalability benchmarks, and user feedback on performance in real-world scenarios.
Q2. How do I assess the scalability of a Big Data Analytics tool?
Test the tool’s ability to handle increasing data volumes, concurrent users, and complex queries without performance degradation.
Q3. What are the key features to look for in a data visualization tool?
Look for interactive dashboards, drill-down capabilities, customizable charts, support for multiple data sources, and collaboration features.
Q4. Can I customize Big Data Analytics tools to suit my specific needs?
Many tools offer customization options through APIs, plugins, scripting, or configuration settings to tailor workflows and analytics processes.
Q5. What are the potential challenges in implementing Big Data Analytics tools?
Challenges may include data integration complexities, skill gaps in data science and analytics, infrastructure requirements, and ensuring data privacy and security.
Conclusion
Choosing the right big data analytics tool requires a thorough assessment of factors such as scalability, performance, integration, cost, and security. By understanding your organization’s needs, evaluating available tools, and considering real-world examples, you can make informed decisions to harness the power of data analytics for actionable insights.
Supercharge Your Data Insights Today with BuzzyBrains!
Unlock the full potential of your data with BuzzyBrains’ advanced analytics solutions. From scalable processing to intuitive visualization, our tools empower organizations to derive meaningful insights and drive strategic decisions. Contact us today to embark on your data-driven journey!