Power of AI and ML: Elevating Data Quality
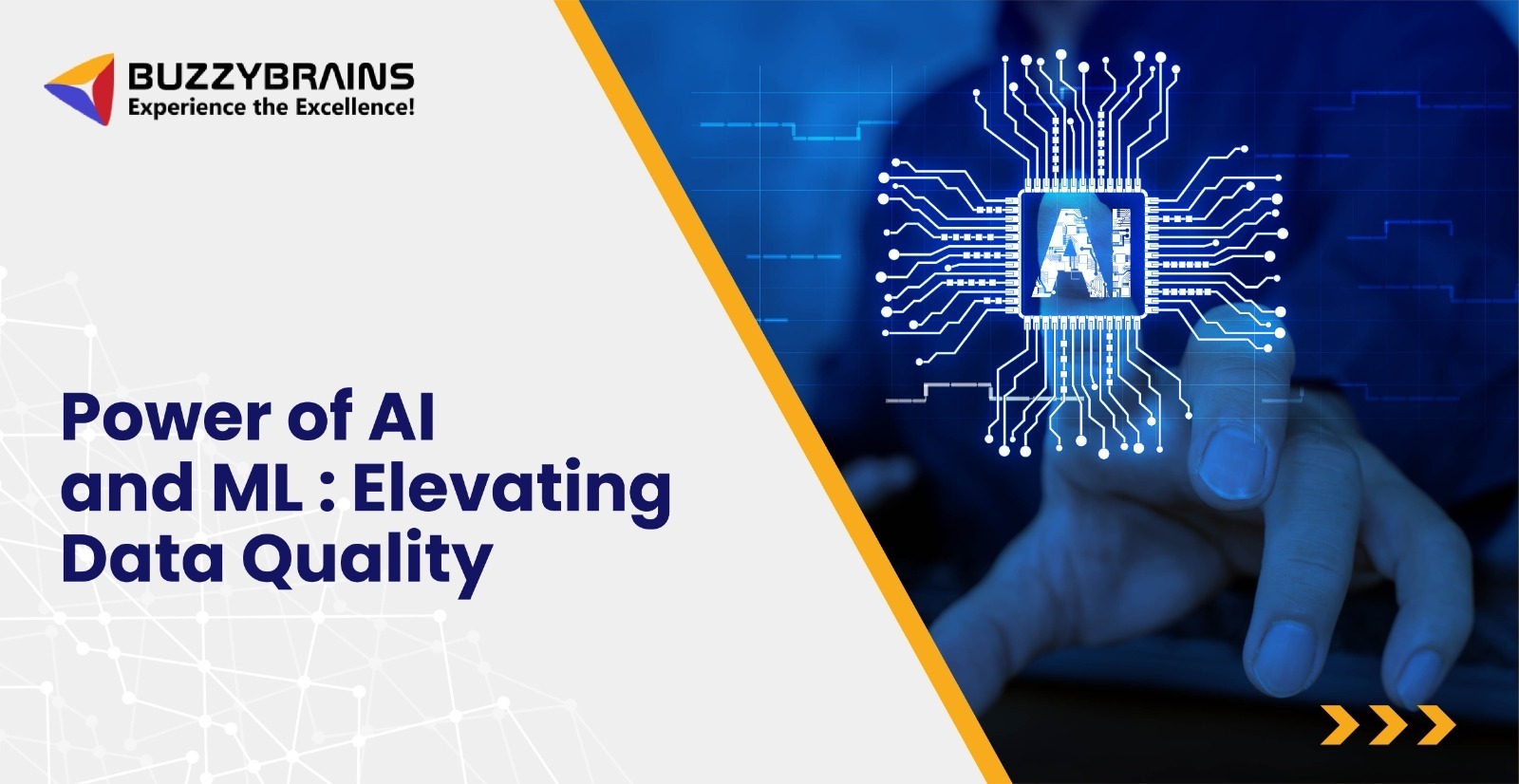
In today’s digital age, data is often referred to as the new oil. However, just like crude oil needs refining to be useful, raw data needs processing and enhancement to unlock its full potential. This is where the power of Artificial Intelligence (AI) and Machine Learning (ML) comes into play, revolutionizing the way businesses handle and improve data quality. In this comprehensive guide, we’ll delve into the intricacies of data quality, explore the challenges associated with maintaining it, understand the pivotal role AI and ML play in enhancing data quality, and discuss future trends in this ever-evolving field.
- What is Data Quality?
- Key Dimensions of Data Quality
- Challenges in Maintaining Data Quality
- Role of AI and ML in Data Quality
- Benefits of Enhanced Data Quality through AI and ML
- Challenges and Considerations
- Future Trends in AI, ML, and Data Quality
- FAQs about AI and ML in Elevating Data Quality
- Conclusion
- Supercharge Your Data Strategy with BuzzyBrains!
What is Data Quality?
Before delving into the role of AI and ML in data quality, it’s crucial to understand what data quality entails. Data quality refers to the accuracy, completeness, consistency, reliability, and relevance of data for its intended use. Essentially, high data quality ensures that the data is fit for purpose and can be relied upon for making informed decisions.
Key Dimensions of Data Quality
- Accuracy: Data accuracy measures how well the data reflects the real-world objects or events it represents.
- Completeness: This dimension assesses whether all required data elements are present.
- Consistency: Consistency ensures that data is uniform and free from discrepancies.
- Reliability: Reliable data is consistent over time and can be trusted for decision-making.
- Relevance: Relevant data is aligned with the specific needs and objectives of the organization.
Challenges in Maintaining Data Quality
Despite the importance of data quality, several challenges can impede its maintenance:
- Data Silos: Disparate data sources can lead to inconsistency and duplication.
- Data Decay: Over time, data can become outdated or inaccurate.
- Data Security: Ensuring data integrity while maintaining security is a constant challenge.
- Data Governance: Lack of clear policies and procedures can result in poor data quality.
- Data Integration: Integrating data from different systems without compromising quality is complex.
- Human Error: Manual data entry and processing can introduce errors.
- Scalability: As data volumes grow, maintaining quality becomes more challenging.
Role of AI and ML in Data Quality
AI and ML play multifaceted roles in enhancing data quality, revolutionizing how organizations manage, analyze, and utilize their data assets. Let’s explore the diverse roles these technologies play:
1. Automated Data Cleansing:
AI-powered algorithms can automate the process of identifying and rectifying data errors, inconsistencies, and duplications. These algorithms continuously learn from data patterns, enabling them to improve data quality over time without manual intervention.
2. Data Enrichment:
ML models excel in data enrichment by analyzing existing datasets, identifying missing information, and filling in gaps. For instance, Natural Language Processing (NLP) models can extract valuable insights from unstructured data sources, enhancing the overall completeness and accuracy of the data.
3. Anomaly Detection:
AI algorithms are adept at detecting anomalies and outliers in data, which may signify data quality issues or potential fraud. By flagging such anomalies, organizations can take corrective actions promptly, maintaining data integrity and reliability.
4. Predictive Maintenance:
ML models can predict data quality issues before they occur by analyzing historical data patterns and trends. This proactive approach enables organizations to pre-emptively address potential data quality challenges, ensuring consistent data quality standards.
5. Data Quality Monitoring:
AI-powered monitoring tools continuously assess key data quality metrics, such as accuracy, completeness, consistency, and reliability. These tools provide real-time insights into data quality status, allowing organizations to promptly address any deviations or discrepancies.
6. Natural Language Processing (NLP):
NLP techniques enhance data quality by extracting meaningful information from textual data sources. Sentiment analysis, entity recognition, and text summarization are examples of NLP applications that contribute to improved data quality and decision-making.
7. Machine Learning Data Profiling:
ML-driven data profiling tools analyze data attributes, distributions, and relationships, uncovering hidden patterns and anomalies. This deep dive into data characteristics helps identify potential data quality issues and opportunities for optimization.
8. Integration with Data Governance:
AI and ML technologies can be integrated into data governance frameworks, automating compliance checks, ensuring data lineage, and enhancing overall data governance practices. This integration promotes data quality consistency and adherence to regulatory standards.
Benefits of Enhanced Data Quality through AI and ML
The integration of AI and ML into data quality processes yields a plethora of benefits, transforming how organizations leverage data for strategic decision-making and operational excellence. Here are the key benefits of enhanced data quality through AI and ML:
1. Improved Decision-Making:
High-quality data, facilitated by AI and ML, leads to more accurate and reliable analysis, enabling informed decision-making across all business functions. Organizations can confidently rely on data-driven insights for strategic planning and execution.
2. Cost Reduction:
Automated data quality processes driven by AI and ML reduce manual effort, minimize human errors, and streamline data cleansing, enrichment, and validation tasks. This automation translates to cost savings and operational efficiency improvements.
3. Enhanced Customer Experience:
Reliable data quality ensures personalized and seamless customer experiences. AI and ML-driven analytics enable organizations to gain deeper insights into customer behavior, preferences, and needs, delivering targeted and relevant services or products.
4. Compliance and Risk Management:
AI-powered data governance tools ensure compliance with regulatory requirements, data privacy standards, and industry best practices. By maintaining data integrity and security, organizations mitigate risks associated with data breaches and regulatory non-compliance.
5. Innovative Insights and Business Agility:
ML-driven data analysis uncovers valuable insights, trends, and correlations that drive innovation and business growth. Organizations can identify new opportunities, optimize processes, and adapt swiftly to changing market dynamics.
6. Operational Efficiency and Scalability:
Streamlined data quality processes, enabled by AI and ML, enhance operational efficiency, scalability, and agility. Organizations can handle growing data volumes, complex data integration challenges, and diverse data sources with ease and accuracy.
7. Real-time Monitoring and Predictive Maintenance:
AI-powered monitoring tools offer real-time insights into data quality metrics, enabling proactive identification and resolution of data quality issues. Predictive maintenance models anticipate potential data quality challenges, minimizing disruptions and downtime.
8. Competitive Advantage:
By leveraging AI and ML for enhanced data quality, organizations gain a competitive edge in the market. They can harness data as a strategic asset, driving innovation, improving customer satisfaction, and outperforming competitors.
In essence, the benefits of enhanced data quality through AI and ML extend beyond operational improvements to strategic advantages, positioning organizations for sustained growth, resilience, and success in a data-driven landscape.
Challenges and Considerations
While AI and ML bring significant advantages to data quality, certain challenges and considerations must be addressed:
Challenges:
- Algorithm Bias: AI models can exhibit bias if not trained on diverse datasets.
- Data Privacy: Ensuring data privacy and security while leveraging AI technologies is critical.
- Integration Complexity: Integrating AI into existing data systems requires careful planning and execution.
- Skill Gap: Organizations may face challenges in acquiring the necessary AI and ML expertise.
- Ethical Considerations: AI-driven decisions must align with ethical standards and societal values.
- Data Governance: Clear data governance policies are essential to mitigate risks associated with AI and ML.
Considerations:
- Data Quality Strategy: Develop a comprehensive strategy for managing data quality throughout its lifecycle.
- AI Ethics Framework: Establish an ethical framework for AI and ML initiatives, focusing on fairness, transparency, and accountability.
- Continuous Learning: Invest in ongoing training and upskilling to keep pace with AI and ML advancements.
- Collaboration: Foster collaboration between data scientists, domain experts, and business stakeholders to ensure AI solutions meet business needs.
- Performance Monitoring: Regularly monitor the performance of AI models to ensure accuracy and reliability.
- Regulatory Compliance: Stay abreast of data regulations and compliance requirements relevant to AI and ML applications.
Future Trends in AI, ML, and Data Quality
Key trends shaping the future of AI, ML, and data quality include:
- Explainable AI: Focus on developing AI models that can explain their decisions and predictions.
- AI-Powered Data Governance: Integration of AI into data governance frameworks for enhanced control and compliance.
- Automated Data Quality Pipelines: End-to-end automation of data quality processes using AI and ML.
- AI-driven Predictive Analytics: Leveraging AI for predictive analytics to anticipate data quality issues.
- Edge AI for Data Quality: Deployment of AI models at the edge for real-time data quality checks.
- Ethical AI Standards: Development of industry-wide standards and guidelines for ethical AI practices.
FAQs about AI and ML in Elevating Data Quality
Q1. How do AI-driven data quality platforms work? AI-driven data quality platforms use algorithms to analyze and cleanse data, identifying errors, inconsistencies, and anomalies. These platforms often incorporate ML models for continuous learning and improvement.
Q2. What are some common data quality issues that AI and ML can address? AI and ML can address common data quality issues such as data duplication, inaccuracies, incompleteness, inconsistency, and outdated information. These technologies can also detect outliers and anomalies that may indicate data quality issues.
Q3. What is data enrichment, and how do ML models enhance datasets? Data enrichment involves enhancing datasets by adding missing information or validating existing data. ML models achieve this by analyzing patterns, trends, and relationships within the data to generate accurate predictions or fill in missing values.
Q4. Can AI and ML be used to predict data quality issues before they occur? Yes, AI and ML can be used for predictive analytics to anticipate data quality issues. By analyzing historical data patterns and trends, AI models can identify potential issues early, enabling proactive intervention to maintain data quality.
Q5. What are some recommended AI and ML tools and software for enhancing data quality? Some recommended AI and ML tools for enhancing data quality include IBM Watson, Informatica Data Quality, Talend Data Fabric, Trifacta, and Alteryx. These platforms offer a range of features for data cleansing, enrichment, anomaly detection, and predictive analytics.
Conclusion
The power of AI and ML in elevating data quality cannot be overstated. These technologies enable organizations to overcome data quality challenges, improve decision-making, enhance customer experiences, and drive innovation. However, successful implementation requires a strategic approach, addressing challenges, considering ethical implications, and staying abreast of future trends. By harnessing the full potential of AI and ML, businesses can supercharge their data strategies and unlock new opportunities for growth and success.
Supercharge Your Data Strategy with BuzzyBrains!
At BuzzyBrains, we specialize in AI-driven solutions that elevate data quality and empower businesses to thrive in the digital era. Our cutting-edge AI and ML technologies streamline data processes, uncover valuable insights, and ensure data reliability and integrity. Contact us today to supercharge your data strategy and unleash the full potential of your data assets.