The Future of AI: How Object Detection is Revolutionizing Industries?
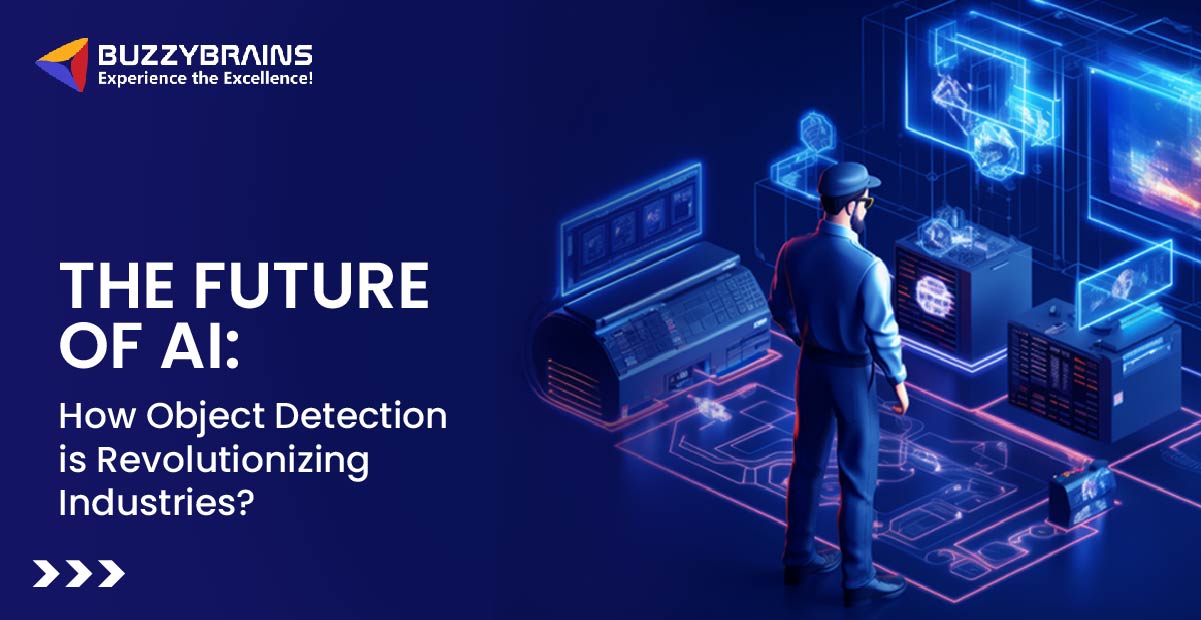
Artificial Intelligence (AI) has been transforming the way industries operate, and one of its most impactful advancements is object detection. This AI-driven technology allows machines to recognize and locate objects in images and videos, opening endless possibilities for innovation.
From enhancing retail operations to improving healthcare diagnostics, object detection is changing the face of multiple industries. In this post, we’ll explore what object detection is, how it works, and how it is shaping the future of AI-driven industries.
- What is Object Detection?
- Methods and Algorithms of Object Detection
- How Object Detection Works?
- How Object Detection is Revolutionizing Industries?
- Benefits of Object Detection Across Different Sectors
- The Role of AI and Deep Learning in Improving Object Detection
- The Future of Object Detection in AI
- Real-World Use Cases of Object Detection
- FAQs About Object Detection
- Conclusion
- Revolutionize Your Business with AI-Powered Object Detection – Partner with BuzzyBrains!
What is Object Detection?
Object detection is a computer vision task that involves identifying and locating objects within images or videos. Unlike simple image recognition, which classifies objects in an image, object detection goes a step further by drawing bounding boxes around the detected objects and identifying their specific locations.
Object detection combines several technologies, including machine learning, deep learning, and neural networks, to achieve high levels of accuracy and performance. As a foundational element of many AI applications, it has become indispensable in fields like autonomous driving, healthcare, security, and manufacturing.
Methods and Algorithms of Object Detection
Object detection can be implemented using different algorithms and architectures, each offering varying levels of accuracy and speed. Below are some of the most used methods or algorithms:
- R-CNN (Region-based Convolutional Neural Networks): Divides the image into regions, runs a CNN on each region, and classifies objects within each region.
- Fast R-CNN: An improvement on R-CNN, Fast R-CNN speeds up the detection process by running CNNs once for all regions.
- Faster R-CNN: Further optimized by using Region Proposal Networks (RPN) to generate regions, improving the speed of object detection.
- YOLO (You Only Look Once): A real-time object detection algorithm that processes the entire image at once, making it much faster than R-CNN-based approaches.
- SSD (Single Shot Multibox Detector): Another real-time method that focuses on detecting objects at multiple scales, balancing speed and accuracy.
Each of these architectures has its advantages and use cases, depending on the application requirements, such as speed, accuracy, and computational power.
How Object Detection Works?
Object detection involves several key steps that allow the technology to identify and classify objects in real time. Here’s a breakdown of how it works:
Step 1: Image Acquisition
The first step is capturing images or video frames from a camera or other imaging device. These images are then processed by the object detection model.
Step 2: Preprocessing the Input Data
Before the data is fed into the AI model, it goes through preprocessing steps like resizing, normalization, and data augmentation to improve detection accuracy.
Step 3: Feature Extraction
The object detection model extracts feature (such as edges, textures, and shapes) from the image using a neural network, which helps in identifying the objects present.
Step 4: Object Classification
Based on the extracted features, the model classifies the objects within the image, determining what the objects are (e.g., a car, person, or dog).
Step 5: Localization and Bounding Box Prediction
The model draws bounding boxes around the detected objects, accurately identifying their location in the image.
Step 6: Post-Processing
After the initial detection, the system refines the results by applying techniques like non-maximum suppression (NMS) to reduce false positives and improve accuracy.
How Object Detection is Revolutionizing Industries?
Object detection is rapidly transforming the way industries function by automating tasks, improving operational efficiency, and enhancing decision-making processes. This AI-driven technology allows machines to “see” and understand the world in ways that were previously unimaginable. By identifying and locating objects within images and videos, object detection is revolutionizing industries in profound ways, leading to more accurate insights, faster operations, and better customer experiences.
Here are some of the key industries revolutionized by object detection:
- Healthcare: Object detection aids in medical imaging, helping detect tumors, fractures, and other anomalies in X-rays, MRIs, and CT scans.
- Retail: Enhances inventory management by automatically detecting stock levels on shelves, powering smart checkout systems, and providing customer insights.
- Manufacturing: Used for automated quality control, object detection can identify defects in products during the production process.
- Autonomous Vehicles: Object detection is essential for self-driving cars to identify pedestrians, other vehicles, and obstacles, ensuring safe navigation.
- Security and Surveillance: Enables real-time detection of suspicious activities, intruders, and even facial recognition for access control.
Object detection is not just limited to these sectors but is gradually expanding into other industries, revolutionizing operational efficiency and safety.
Benefits of Object Detection Across Different Sectors
Object detection offers numerous benefits to industries by automating processes, improving safety, and reducing costs. Some key benefits include:
- Automation: Reduces the need for human intervention in tasks like quality control, inventory management, and surveillance.
- Cost Savings: Lowers operational costs by automating tasks that were previously labor-intensive.
- Improved Accuracy: Provides precise detection and classification of objects, reducing errors in critical tasks like medical diagnostics or product inspection.
- Enhanced Safety: Helps autonomous systems (like cars or drones) avoid accidents by detecting obstacles in real time.
- Better Decision Making: Provides businesses with actionable insights by analyzing detected objects and patterns over time.
The Role of AI and Deep Learning in Improving Object Detection
AI and deep learning have played a pivotal role in advancing object detection technology. Early object detection methods relied heavily on manual feature extraction, but with the advent of deep learning, models can now automatically learn complex features from data, making detection faster and more accurate.
Some of the key technologies and frameworks driving these advancements include:
- TensorFlow: An open-source deep learning framework widely used for building object detection models.
- PyTorch: Another popular framework that provides flexibility and ease of use for training complex AI models.
- YOLO (You Only Look Once): A real-time object detection model that offers high-speed detection without compromising accuracy.
- R-CNN and Faster R-CNN: Region-based CNN models that improve the precision of object detection, particularly in cases where speed is not the primary concern.
With continuous improvements in neural networks and AI frameworks, object detection is becoming more efficient, scalable, and capable of handling complex real-world tasks.
The Future of Object Detection in AI
As AI technologies continue to advance, the future of object detection looks promising. Here are some trends to look forward to in the coming years:
- Edge Computing: Running object detection models on edge devices (like smartphones and IoT devices) to enable real-time processing without relying on cloud infrastructure.
- 5G and IoT Integration: With faster network speeds and interconnected devices, object detection will become more integrated into everyday tasks, like smart homes or cities.
- AI-Powered Robotics: Object detection will continue to fuel the development of autonomous robots that can navigate and interact with the physical world.
- Improved Real-Time Analytics: Combining object detection with advanced analytics to allow businesses to make instant, data-driven decisions.
- Ethical AI and Data Privacy: As object detection becomes more widespread, there will be growing attention on ethical use, particularly in areas like surveillance and data privacy.
These trends will further solidify the role of object detection in revolutionizing industries, making it a critical component of the AI landscape.
Real-World Use Cases of Object Detection
Object detection has already been deployed in various industries, with some notable real-world use cases:
- Healthcare: AI-powered object detection is improving the accuracy of diagnosing diseases like cancer by analyzing medical images and identifying abnormalities.
- Autonomous Vehicles: Self-driving cars rely on object detection to recognize pedestrians, traffic signs, and obstacles, ensuring safer navigation.
- Retail: Smart checkout systems in retail use object detection to recognize products without the need for barcodes, making shopping faster and more efficient.
- Security: Surveillance systems use object detection to detect unusual behaviors, such as trespassing or suspicious movements, in real time.
- Agriculture: Farmers use drones equipped with object detection to monitor crop health, identify pests, and optimize irrigation.
These applications demonstrate the versatility of object detection across different fields, showing how it can solve a wide range of business challenges.
FAQs About Object Detection
Q1. What industries are currently using object detection the most?
Industries like healthcare, retail, manufacturing, autonomous vehicles, and security are currently leveraging object detection the most.
Q2. How does object detection differ from image recognition?
Image recognition identifies what an object is, while object detection not only identifies the object but also locates it within the image by drawing a bounding box around it.
Q3. Can object detection work in low-light or extreme conditions?
Yes, with advanced techniques like infrared imaging or thermal cameras, object detection can work in low-light or extreme conditions.
Q4. What are the limitations of current object detection models?
Current object detection models may struggle with occlusion (when objects are partially hidden), variations in lighting, and false positives or negatives in challenging environments.
Q5. What kind of data is required to train an object detection model?
Object detection models require large datasets of labeled images or video frames with annotations marking the objects and their corresponding bounding boxes.
Conclusion
Object detection is not just a technical achievement in AI; it is a groundbreaking technology transforming industries worldwide. From making healthcare diagnostics more accurate to enhancing autonomous driving and retail operations, its applications are vast and varied. As AI continues to evolve, so will object detection, bringing new opportunities and challenges. For businesses looking to stay ahead, investing in object detection and AI technologies is no longer optional but necessary.
Revolutionize Your Business with AI-Powered Object Detection – Partner with BuzzyBrains!
At BuzzyBrains, we specialize in delivering cutting-edge AI solutions, including advanced object detection systems tailored to your business needs. Whether you’re in healthcare, retail, manufacturing, or any other industry, we can help you harness the power of AI to revolutionize your operations. Contact us today to explore how object detection can transform your business!